What Innovative Uses of Artificial Intelligence Have Impacted Cybersecurity?
In the rapidly evolving landscape of cybersecurity, artificial intelligence is playing a pivotal role. We've gathered insights from a Chief Technology Officer and an AI Solutions Specialist, among others, on the innovative uses of AI in this field. From identifying AI as a security risk to AI-assisted code scanning that reduces errors, explore six groundbreaking ways AI is impacting cybersecurity.
- Identifying AI as a Security Risk
- AI for Holistic Security Strategy
- AI Detects Anomalies and Threats
- Deepfakes: A New AI Cybersecurity Threat
- AI Predicts and Thwarts Cyber Threats
- AI-Assisted Code Scanning Reduces Errors
Identifying AI as a Security Risk
System architects are just waking up to the LLMs themselves as security vulnerabilities; for example, they may have anonymized personally identifiable information (PII), but LLM pattern recognition is capable of de-anonymizing data and turning it back into PII. This change of state is a novel vulnerability.
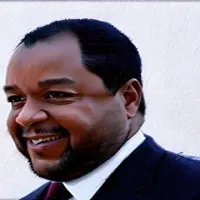
AI for Holistic Security Strategy
Traditional methods of addressing security threats often fall short because they rely on isolated, specific solutions that do not offer a holistic approach. These solutions operate independently and fail to provide a comprehensive security strategy. By employing AI to learn from an organization's daily activities and integrating context from a variety of internal and external sources, such as email, cloud, networks, and many more, we can develop a more complete and effective security approach.
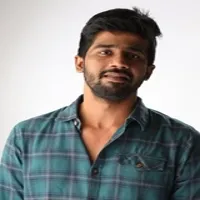
AI Detects Anomalies and Threats
One innovative use of AI in cybersecurity is detecting anomalies and potential threats. AI systems use machine-learning models to analyze network traffic and user behavior data. They identify patterns that deviate from the norm. This allows the systems to alert security teams about potential threats. Teams can then investigate further. This proactive approach improves threat-detection capabilities. It enables faster response times, too. Overall, it enhances an organization's security posture.
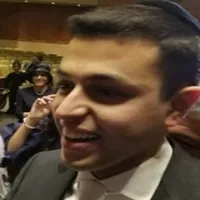
Deepfakes: A New AI Cybersecurity Threat
One innovative use of artificial intelligence in cybersecurity I've seen is the creation of User-Generated Content (UGC) deepfakes.
Scammers are using AI to generate deepfake videos of UGC creators and everyday people, which are then used in scams.
A recent high-profile case involved a finance worker who lost $25 million after being deceived by a deepfake video of their company's Chief Financial Officer (CFO). This AI-UGC situation highlights the potential dangers of AI misuse in cybersecurity and underscores the need for better security measures to detect and counteract these threats.
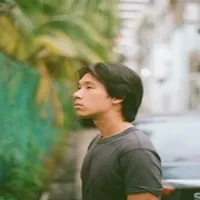
AI Predicts and Thwarts Cyber Threats
Artificial intelligence is revolutionizing cybersecurity through predictive threat intelligence. By analyzing real-time data, AI can spot patterns and anomalies, foreseeing cyber threats before they strike.
For instance, a financial services firm employed AI to catch signs of a complex phishing attack early on. This proactive approach helped them thwart the threat, preventing a damaging data breach. Not only does this safeguard sensitive data, but it also boosts efficiency by cutting down on response times and resource allocation.
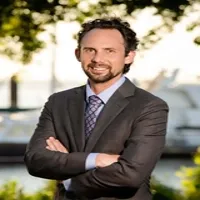
AI-Assisted Code Scanning Reduces Errors
I've seen some organizations use artificial intelligence for AI-assisted code scanning. Traditionally, SAST (Static Application Security Testing) uses a "sources and sinks" approach to scan code. This method tracks the flow of data to identify common security pitfalls. While effective, this approach often results in numerous false positives, which then require manual validation.
The integration of AI and machine learning can add significant value here by learning the context or intent around potential issues in the codebase, which helps reduce both false positives and false negatives. Both SAST tools and AI assistants have also been integrated directly into code editors. This allows developers to catch errors before the code is even submitted. There are some limitations, such as language support and scalability issues with very large codebases, but these challenges are being quickly addressed.
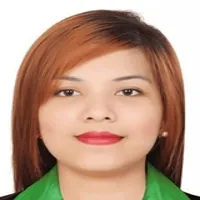